There's been a lot of talk lately about whether AI lives up to the lofty expectations it set initially or if it's just hype. Some argue that the AI bubble has already burst or is about to collapse. Views on this range greatly, from direct criticism to more in-depth, subtle evaluations.
I recently finished reading Benedict Evans' extensive piece, "The AI Summer," which offers a perceptive analysis and is recommended reading for anybody with an interest in AI.
Evans points out that a lot of people experimented with ChatGPT and other generative AI apps but abandoned them quickly. Additionally, he makes the point that although a lot of businesses have started trial programs, there hasn't been much mainstream acceptance.
In recent years, both the tech industry and the general public have focused a great deal of attention on creative AI. Although ChatGPT and other AI-based apps became massively popular within a few months, their usage growth has recently stalled.
Evans believes that while Large Language Models (LLMs) are a powerful new technology, turning them into practical, everyday business tools will take more time. Currently, a significant amount of investment is going into developing creative AI technology and models, but it's unclear how these investments will pay off.
So, does Evans have a point? Is AI development really slowing down, and is the bubble about to burst?
Is Growth Stagnating?
Looking at the numbers, it's clear that creative AI has been growing at a breakneck pace. For instance, ChatGPT reached 100 million users in just two months – a speed unprecedented by any other technology.
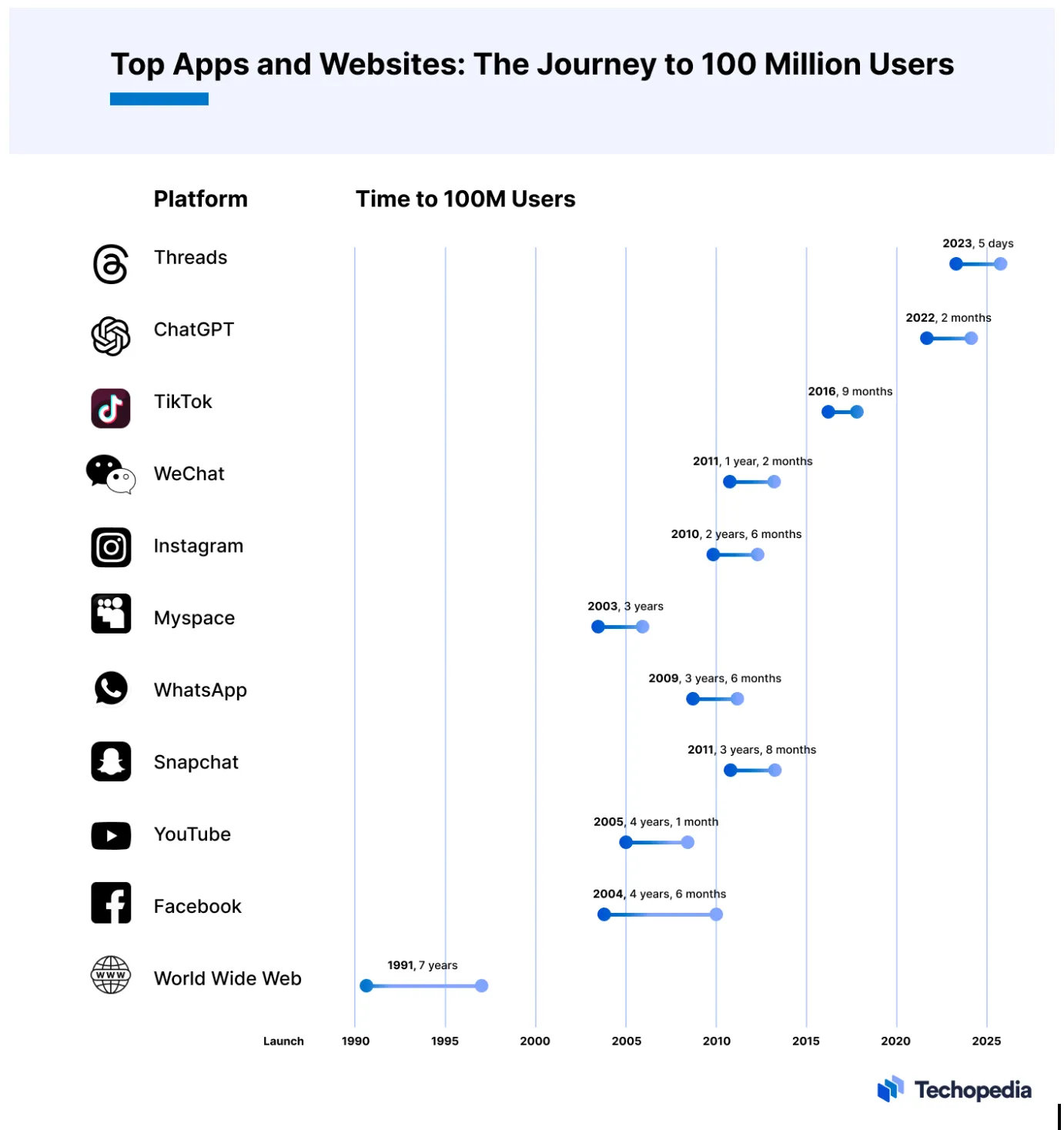
Allikas: Tehchopedia
However, ChatGPT's initial rapid growth hasn't seen the expected continuation. According to Similarweb's website traffic statistics, after a sharp rise in January 2023, ChatGPT's usage has remained relatively stable throughout the year, hovering around 1.5 to 1.7 billion monthly visits. It wasn't until May of this year that it managed to grow again, surpassing 2.5 billion users for the first time.
Does this indicate that growth has entirely stalled? I'm not so sure. Firstly, ChatGPT's growth has already shown signs of recovery. Additionally, new strong competitors like Claude and Gemini have entered the market, each capturing their share.
Moreover, more and more people are integrating AI tools into their daily work. Various studies, including those cited by Evans from the Pew Research Center, indicate a clear increase in ChatGPT's use in the workplace.
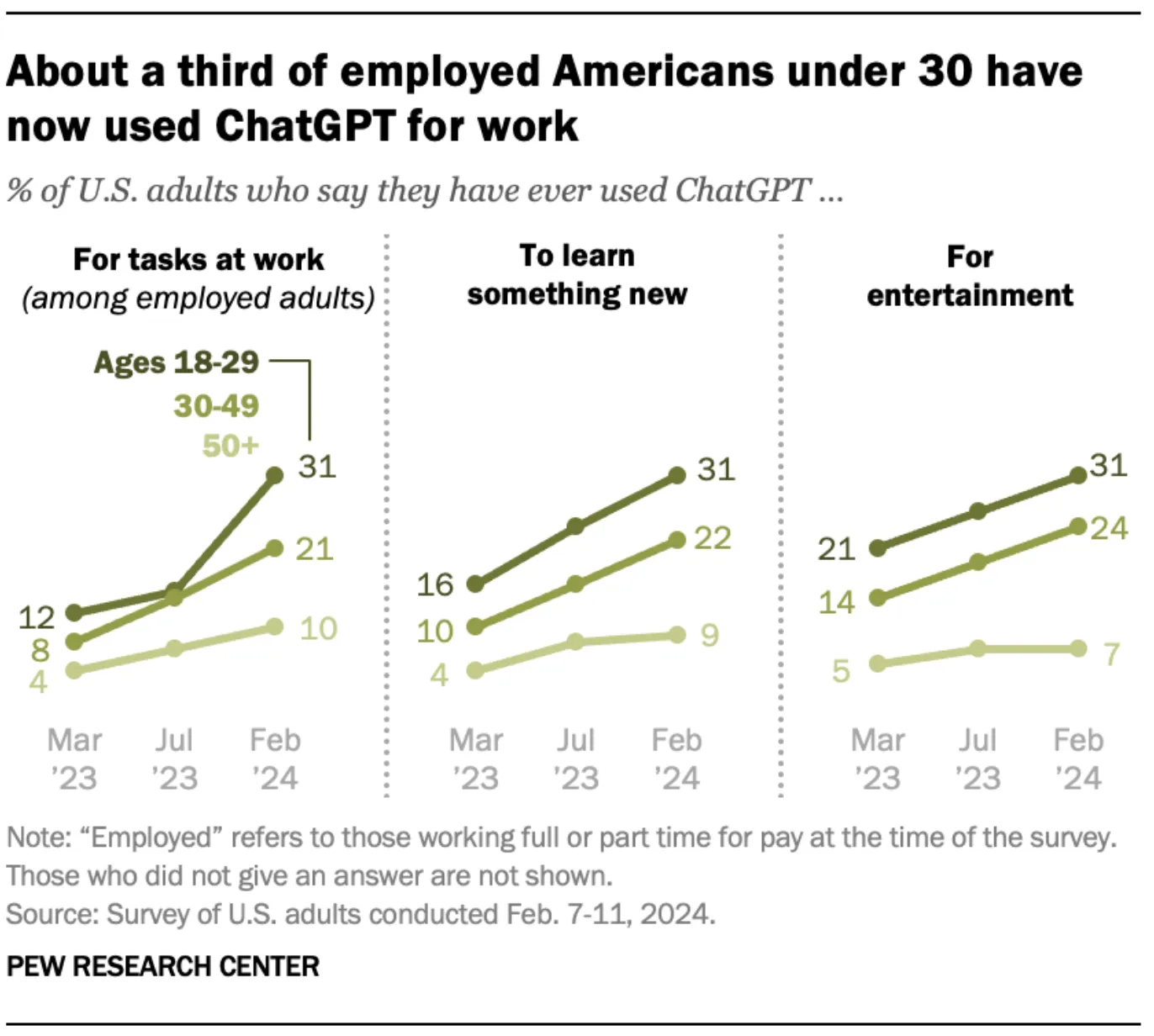
I'm inclined to agree that one potential barrier to the broader adoption of AI-based tools is the complexity of integrating them into existing workflows. This process often requires resources, new skills, and dedication.
But it would be incorrect to claim that ChatGPT's growth has completely dried up and that most people try ChatGPT only to forget about it soon after.
Experimentation vs. Adoption
While many companies have started initial experiments with creative AI, only a few have reached systematic and widespread use of AI within their organizations. Most companies are still in the piloting and experimenting phase, exploring the technology's potential and limitations.
There is also a sense of caution among companies, partly due to the novelty of the technology and sometimes because of the uncertainty among executives about its effectiveness. Many leaders are waiting for more definite ROI and clear evidence of AI's effectiveness before committing significant investments to full-scale solutions.
One possible hurdle is customizing AI solutions to meet specific company needs and workflows. Every company has its unique structure, culture, and processes, making it difficult to find a one-size-fits-all AI solution.
Thus, adopting AI often requires a tailored approach and close collaboration between technology developers and end users.
Gen AI: Technology or Product?
Another issue is that we are still in the technology development phase, and the right products have yet to hit the market.
While LLMs are a new and powerful technology, it's evident that transforming them into fully-fledged, useful products for everyday business operations takes time. For example, ChatGPT might seem like a finished product, but it's more of a "minimum viable product" (MVP) that meets basic needs quickly.
For companies, a significant challenge is integrating AI into specific workflows. There was long-standing uncertainty about how OpenAI handled data input into ChatGPT, but recent months have brought more clarity. However, it's understandable if not all CTOs are up to date with OpenAI's latest updates.
Additionally, OpenAI seems undecided on whether to continue developing ChatGPT for business users' needs or as a chatbot for individual users (similar to Siri or Alexa).
Although LLMs have automated many tasks like text generation and analysis, their application for certain business needs remains limited. In many fields, LLM-based solutions are not yet reliable or accurate enough to be used without human oversight. There are areas where the margin for error is critical, and even the smallest mistakes are unacceptable.
Developing the technology is one aspect, but user experience and interface design are also crucial. LLMs may offer powerful capabilities, but their real value depends significantly on how well they are integrated into user-friendly tools.
Where Does the Money Go, and From Where It Comes Back?
Benedict Evans raises the issue of investment returns and warns of a potential bubble. A leading venture capital firm in the U.S., Sequoia, also highlighted this concern in a blog post. The development of generative AI has attracted immense attention and investment. Currently, a lot of investment is going into developing creative AI technology and models, with less focus on practical application in businesses.
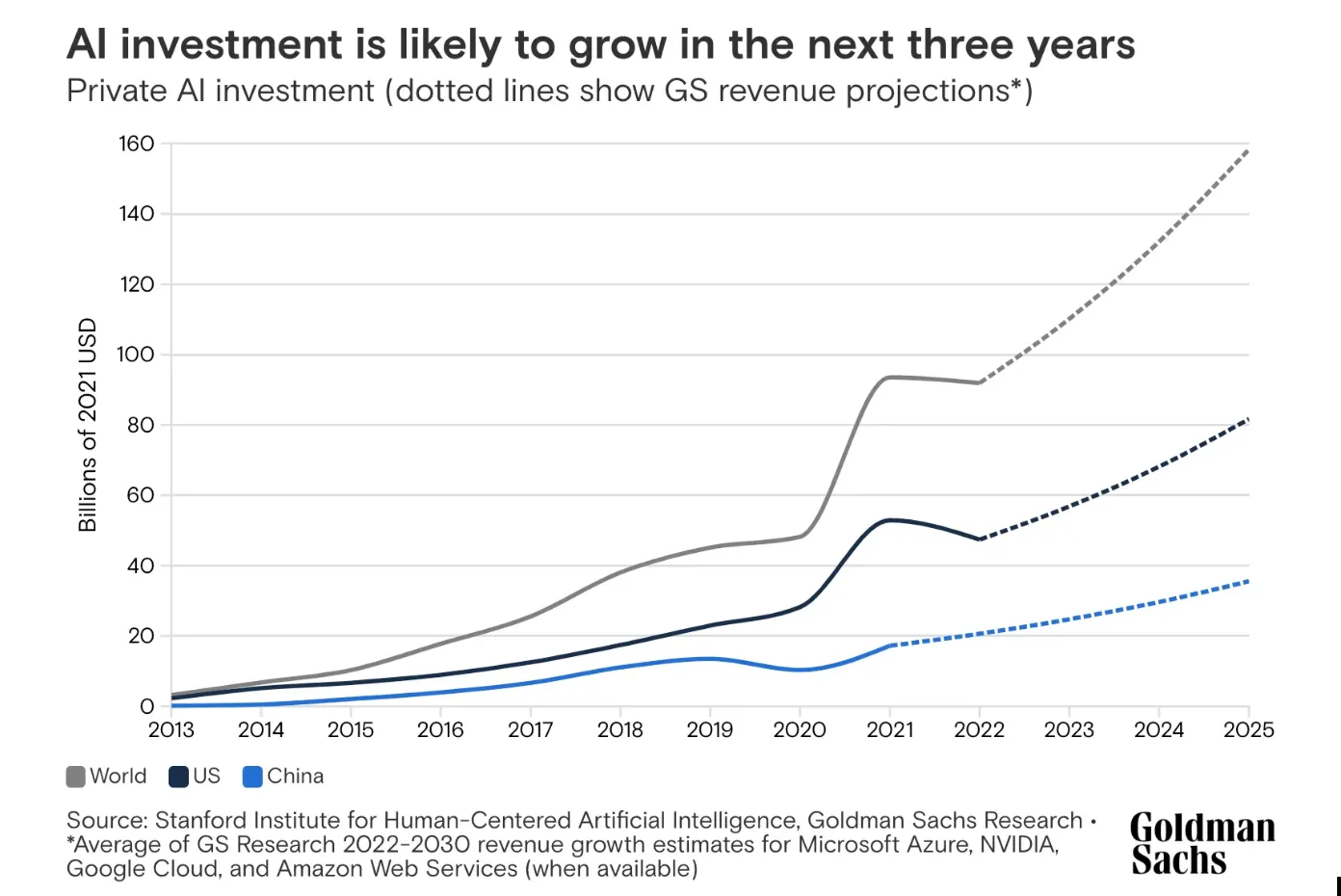
However, the absolute amount of money being invested in AI is enormous. It is essential to consider that large investments always come with risks associated with bubbles and overinvestment. History shows that technological bubbles can form when expectations and reality don't align.
If companies and investors expect rapid and dramatic changes from AI, they might be disappointed if the results don't match their expectations quickly.
In my view, although a lot of venture capital has flowed into AI in the past two years and the investment sums are incredibly large, the situation is not yet comparable to the dot-com boom.
It's important to remember that the largest investments are currently being led by big tech companies in strong financial positions, such as Microsoft, Google, and Meta. Their financial situation and investment practices differ significantly from venture capital firms, mainly in terms of investment period length, as they are willing to wait longer for returns on their investments than typical venture funds.
One thing is clear: besides NVIDIA, various consultants are also making good money right now. For example, Boston Consulting Group (BCG) predicts that 20% of their revenues this year will come from advising large companies on generative AI, indicating that many companies are still in the planning and research phase. Consultants' roles have become increasingly important as companies seek guidance on effectively integrating AI into their operations.
What Else to Consider?
Having spent some time training and consulting various companies in the AI field, I find that AI is not a magic solution but a specific software tool that still requires experimentation and testing. While many tasks can already be accelerated and processes made more efficient, the ultimate potential will likely be realized in a few years.
Currently, ChatGPT is not the easiest tool to integrate into daily work seamlessly. Using it requires adjustment, learning, and experimentation. Employees might not immediately grasp all the possibilities ChatGPT offers.
Therefore, it's crucial to give employees the opportunity to experiment with ChatGPT and other AI tools to develop an initial understanding of the specific models' capabilities. Additionally, companies should systematically consolidate best practices and standardize AI solutions based on these practices. One approach could be creating CustomGPTs within ChatGPT based on the best prompts.
Moreover, conducting an audit of current information systems and analyzing which processes they handle today and where AI could take over tasks currently performed by humans is essential.
Establishing a systematic methodology for evaluating and testing AI solutions is also crucial to measure which prompts are the most stable and provide the best results. We are still searching for the best applications and working methods, making measuring AI utility or ROI quite challenging.
At the same time, AI can accomplish tasks whose ROI is inherently difficult to measure – for example, how do you quantify the benefits of a brainstorming session conducted with ChatGPT?
This highlights the importance of considering multiple factors when companies start integrating generative AI into their systems.
Conclusion
In conclusion, we are at the beginning of adopting generative AI, and in many ways, we are still naive about AI. Although we already see impressive results, it is clear that reaching final outcomes and widespread adoption will take more time.
AI technology-based solutions still need to evolve and adapt to offer real and lasting value. Companies must continue experimenting, learning, and adapting to find the best ways to use AI in their operations.
In other words, the initial hype is over, or at least nearing its end, and now the real journey begins in this field. 😊