Deep within human nature lies a fear of the unknown. It's our survival instinct, which has been with us since the beginning of time, protecting us from potential dangers while preserving what has kept us alive so far. But how does this instinct for self-preservation affect our ability to embrace innovation? Especially now, as we witness one of the greatest technological revolutions of our lifetime - the implementation of artificial intelligence in organizations. People worry that AI might threaten their jobs, make their daily tasks more complicated, or even replace their current tools with something completely unfamiliar. Naturally, this creates resistance.
"The thing with these changes is that nobody really likes them. I'd prefer it if changes were managed so that others change, but I stay the same," joked a good friend when the topic turned to AI adoption in companies. This friendly joke, however, reflects a deep truth: people often prefer their comfort zone, where everything is under control and familiar.
The key to successful change management is understanding that fears and resistance are natural and must be addressed by the entire organization, including management, through practical solutions and support. Attitudes towards change can also be influenced by previous experiences with technological innovations.
A lack of prior work experience with radically new types of technology can increase resistance. Many organizations are accustomed to dealing with small, gradually introduced new tools and methods. This places a great responsibility on the shoulders of leaders: to cope not only with technical changes but also with the challenge of transforming organizational culture.
To reduce resistance to change and alleviate fears, active and open communication is necessary, along with clear plans where all employees have the opportunity to participate and contribute. Transparent processes, consistent feedback, and practical training are key activities that help build trust and readiness in the change management process.
The Status Quo: How Things Have Been So Far
When we think about office work, it's clear that much of it relies on tools and techniques we've been using for over two decades. Email, Excel, Word, and web browsers have been our go-to tools since the late '90s.
It's easy to forget that when these tools were first introduced, adopting them was a big change. When email first entered the workplace, everyone needed training on how to use it. The same was true for the more complex programs like Excel and Word.
For years, the office work routine has remained relatively stable. While we've seen many minor updates and improvements, these have mostly been incremental enhancements to existing tools. Excel gets new features, email security is bolstered, but the core experience remains the same. Most of these changes have required some adjustment and adaptation, but not complete retraining or total overhauls of work practices.
Major technological leaps that completely transform our work style or methods have been few and far between. Perhaps the most significant change in recent memory was the forced shift to remote work due to Covid-19.
This general stability has shaped attitudes, leading many to view our tools as fixed and reliable. As a result, we've grown cautious, even sceptical, about the need for sweeping changes.
Today's Challenges in Implementing AI in Organizations
Implementing artificial intelligence in an organization is a challenge that requires a well-thought-out approach. Leaders responsible for AI adoption in their organizations face a range of tasks, some more complex and multifaceted than others.
But what are these specific challenges, and how can they be best overcome?
Lack of knowledge and experience
Many leaders, accustomed to small, gradual technological innovations, find themselves facing a new reality: AI implementation is much more complex and far-reaching. As AI is a relatively new and constantly evolving field, many leaders lack personal knowledge and experience in how to effectively deploy AI. Understandably, this can create uncertainty and fear of making wrong decisions.
Changing organizational culture
Integrating AI also means changing organizational culture, which is often the biggest and most difficult challenge. Employees must adopt new tools, learn new skills, and adapt to new work processes. Managing change at a cultural level requires the ability to engage and motivate employees so that they feel part of the innovation process.
Resistance to change
People have a natural tendency to resist change, especially when it affects their daily work processes and habits. Leaders must take this into account and develop strategies to mitigate this resistance. Proper communication, openness, and participation throughout the change management process are key factors here.
Technical complexity
Implementing AI technologies often requires specific technical knowledge and skills. Organizations may lack the necessary internal competence and often have to turn to external experts. This can make the process more complex and costly, and there is also a need to manage various third parties and coordinate their activities.
Ethical and legal considerations
The use of AI also involves several ethical and legal issues that leaders must consider. For example, data protection and privacy, AI hallucinations, and bias. These topics require careful consideration and the establishment of appropriate policies and procedures.
Proving ROI
Every change must be economically justified. Leaders must prove that AI implementation brings real benefits to the organization and is a worthwhile investment. This can involve complex ROI calculations and the design of metrics in a situation where there are no established standard practices and few case studies to rely on.
Short-term operational issues
Even well-thought-out and planned AI implementation can cause operational disruptions in the short term. This can be due to both technical problems and increased learning load and adaptation needs for employees.
Keeping these challenges in mind, leaders are in a unique position to successfully guide their organization through the AI integration process, provided they are willing to learn, adapt, and actively participate throughout the change process.
An Example of Today's Problems: The BYOAI Trend

One of the recent notable trends in the adoption of artificial intelligence is "Bring Your Own AI" or BYOAI. This refers to a situation where employees bring their own AI tools and solutions into the company to perform their work tasks. According to the 2024 Annual Work Trend Index, 78% of employees use their own personal AI tools, not the ones provided by their organization. While this may seem like an innovative and proactive step by employees, it comes with several problems and challenges that management must address.
Lack of a unified approach
BYOAI often leads to a situation where different AI tools and solutions are used that are not coordinated or standardized with each other. This can cause data sharing and interoperability issues, as well as hinder the implementation of organization-wide strategies. If each employee uses a different AI solution, confusion and inconsistency in work processes can also arise.
Security risks
Each AI tool needs to be given access to certain data and systems, which means potential security risks. If employees use their own tools, they may not meet the company's security requirements or be sufficiently protected against cybersecurity threats. This can lead to data leaks, cyberattacks, or other security threats that jeopardize the organization's integrity and credibility.
Compliance and regulatory requirements
The use of different AI tools can also create problems in terms of compliance with regulatory requirements. Different solutions may follow different privacy and data protection standards, which can lead to a situation where the company unknowingly violates applicable laws. Meeting regulatory requirements and ensuring compliance requires clear guidelines and control mechanisms, which are difficult to ensure in the context of BYOAI.
Difficulty measuring effectiveness
If employees use different AI solutions, it can be difficult to measure the effectiveness and impact of these tools on the organization. This makes it harder for leaders to assess ROI and can lead to wrong decisions in the future. Standardized solutions allow for better oversight and analysis, which helps leaders make informed decisions.
Training and support
BYOAI brings with it the challenge of training and support. Each AI tool needs to be provided with appropriate training and support, which increases the burden and resource expenditure of the organization. A unified solution would make it much easier and more efficient to organize centralized training and provide support tailored to the needs of employees.
Lack of coordination in innovation and development
The adoption of different AI solutions can hinder the coordination of innovation and development within the organization. If each employee works with their own solution, it can be difficult to leverage collective knowledge and experience that could help develop and improve AI technologies together. A lack of unity and collaboration can slow down innovation and lead to a waste of resources.
The BYOAI trend, as a grassroots initiative, is welcome, but it is also important for management to adopt clear policies and guidelines on the use of AI tools while being open to employee ideas and suggestions. If leaders can strike a balance between an individualized approach and organizational needs, AI adoption can be more successful and efficient.
Change Management Frameworks
The challenges associated with the BYOAI trend underscore the importance of a structured approach to change management when implementing AI in organizations. To address these challenges and ensure an effective AI adoption process, organizations can turn to established change management frameworks.
Change management is a strategic process through which organizations implement changes to achieve desired outcomes. Implementing artificial intelligence represents a significant technological and cultural change, which is why it's important to use established and effective change management frameworks.
Below is the selection of the main change management frameworks that can be applied in the context of AI.
Kotter's 8-Step Change Management Model

John Kotter's change management model is one of the most well-known and widely used frameworks. The model consists of eight steps that help structure and manage changes in an organization:
- Create a sense of urgency: generate motivation for change by emphasizing the importance and benefits of the new technology.
- Build a guiding coalition: form a team to lead the change, including key individuals from different areas.
- Develop a vision and strategy: define a specific vision and strategy for AI implementation to guide and support the changes.
- Communicate the vision: convey your vision to the organization's employees using various communication channels.
- Empower action: remove obstacles to change and provide employees with access to necessary resources and training.
- Generate short-term wins: recognize the achievement of interim goals to motivate employees.
- Sustain acceleration: use successes to drive further changes.
- Institute change: ensure that new practices and technologies become part of the organizational culture and standard working methods.
ADKAR Model
ADKAR is a goal-oriented change management model developed by Prosci. It consists of five elements:
- Awareness: create awareness of the need for change.
- Desire: motivate people to implement and support the changes.
- Knowledge: provide employees with the necessary knowledge and skills to implement the changes.
- Ability: ensure that employees can practically use the new methods and technologies.
- Reinforcement: ensure that changes are permanently reinforced by providing ongoing support and oversight.
Lewin's 3-Stage Model
Kurt Lewini 3-etapiline mudel on üks varasemaid muudatuste juhtimise teooriaid, mis sobib hästi ka AI juurutamiseks. See koosneb kolmest etapist:
- Unfreeze (Sulata lahti): valmistu muudatusteks, loobudes vanadest töömeetoditest ja avades organisatsiooni uutele ideedele ja tehnoloogiatele.
- Change (Muuda): vii läbi muudatused, juurutades uusi tehnoloogiaid ja töömeetodeid.
- Refreeze (Kinnita muutused): stabiliseeri muudatused ja integreeri need organisatsiooni igapäevatöösse.
Bridges' Transition Model
William Bridges' model focuses on people's emotional and psychological transition during change. It consists of three stages:
- Ending: acknowledge and come to terms with the loss of the old and familiar way of working.
- Neutral zone: be prepared for a period of uncertainty and confusion as employees adapt to new tools and processes.
- New beginning: implement and reinforce the new way of working, encouraging and supporting employees in using the new methods.
McKinsey 7S Framework

The McKinsey 7S framework is a holistic approach that takes into account the different components of an organization. This framework helps organizations align their strategy and structure with the changes required by AI technologies:
- Strategy: develop a clear and specific strategy for AI implementation that links the technology to the organization's goals.
- Structure: adapt the organizational structure to support the adoption of AI technologies.
- Systems: update and integrate existing systems and processes to facilitate the use of AI solutions.
- Shared Values: steer the organizational culture and values towards the adoption of AI technologies.
- Style: change the leadership style to foster innovation and change.
- Staff: train and develop employees to possess the necessary skills for using AI technologies.
- Skills: focus on developing human capital and acquiring the necessary skills to ensure that AI applications are used efficiently and to their fullest potential.
***
Other Tips for Change Management in the Context of AI
- Communicate consistently and transparently: Effective communication is key to the success of change management. Explain why changes are necessary, what benefits they bring, and how they affect daily work.
- Involve all levels: Change management should involve all levels of the organization, from management to frontline employees. This creates a sense of unity and promotes collective adoption.
- Provide ongoing training and support: Ensure employees have access to continuous training and support systems so they are prepared to cope with changes and adopt new technologies.
- Measure and analyze results: Monitor the impact and outcomes of changes to get feedback and make corrective steps if necessary. Start with smaller experiments to reduce risks and encourage learning.
Change management frameworks offer a structured approach that helps organizations successfully implement major technological changes such as AI adoption. Choosing the right framework and adapting it to the organization's needs is crucial for the successful and effective implementation of AI.
Practical Recommendations: Lean Approach and Experimentation
In addition to utilizing change management frameworks, organizations can benefit from adopting a Lean approach and conducting experiments when implementing AI. These strategies allow organizations to reduce risks and make informed decisions throughout the complex process of AI adoption
The Lean approach, originally created for manufacturing companies, focuses on creating value through resource conservation and efficiency improvement.
Important Lean IT principles are:
- Focus on value: Concentrate on solutions that create direct value for your organization and customers. Ask yourself how a specific AI application helps solve a real problem or improve a process.
- Holistic value stream: Map and analyze the entire process to understand where and how AI can create added value. Remove all activities that do not add value.
- Pull principle: Manage changes according to demand, not supply. Implement AI solutions gradually and on an as-needed basis.
- Continuous improvement: Foster a culture of continuous improvement where every employee is involved in seeking innovations and improvements.
These principles can be successfully applied in the context of AI implementation. Using Lean methodologies helps avoid unnecessary costs and increase the effectiveness of changes.
Drawing inspiration from Lean IT principles, we propose a structured approach for AI implementation that consists of the following key steps:
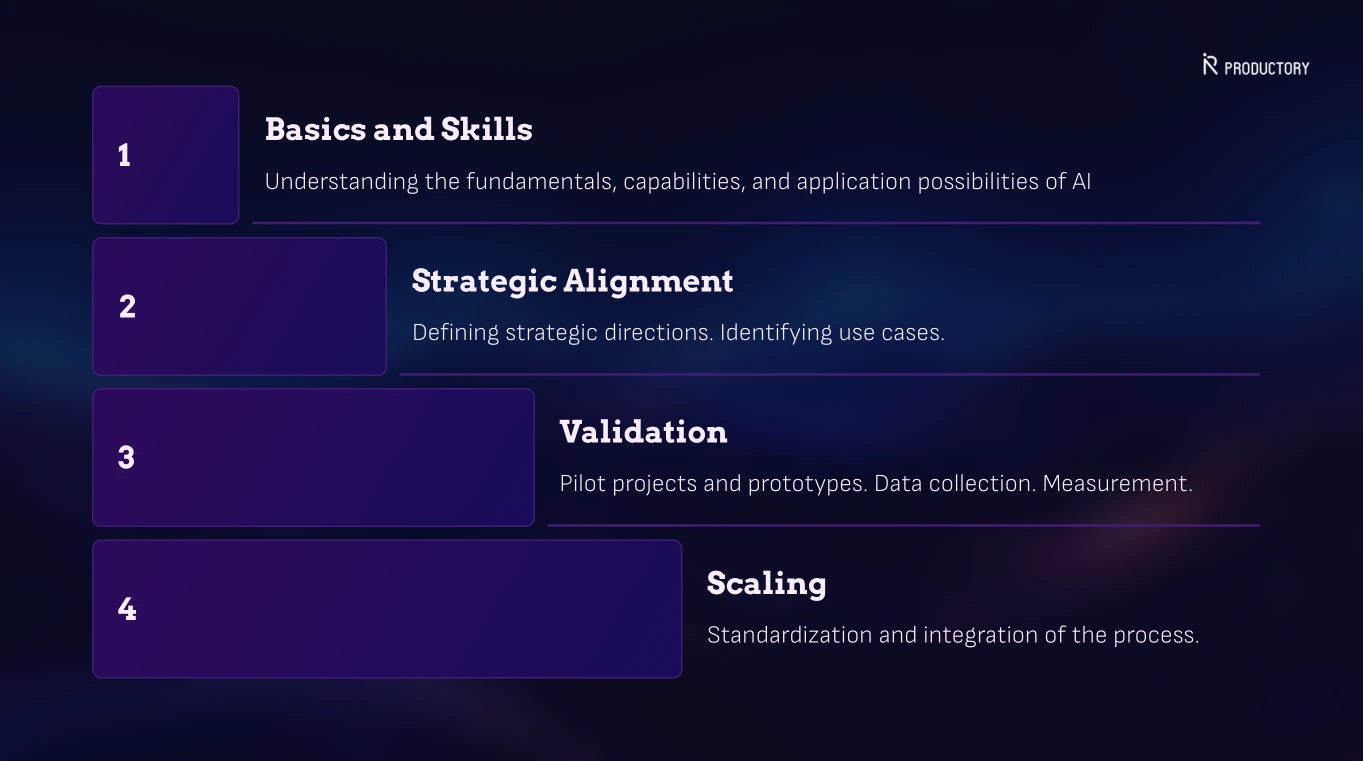
Basic Knowledge and Skills
Lean focuses first on training and developing people, as a knowledgeable and skilled workforce can maximize the benefits of innovation.
- AI fundamentals: Organize training sessions where employees learn AI principles and become familiar with implementation possibilities. Lean IT recommends using short and practical training sessions that focus on real-world applications and business value.
- AI capabilities and opportunities: Organize workshops or hackathons that help understand how AI solutions can be applied in everyday work. According to the Lean IT methodology, the focus should be on quickly creating value and avoiding excessive complexity.
Strategic Alignment
Lean emphasizes strategic planning and targeted activities to ensure resource efficiency and maximum impact.
- Defining strategic directions: Treat AI implementation in the context of the organization's broader strategic goals. The focus should be on how AI helps optimize existing processes and increase value creation for the customer.
- Identifying use cases: Choose the most important and valuable use cases where AI could bring benefits. We recommend starting with simple projects that create immediate value to quickly see results and build trust.
Validation
Pilot projects and small-scale experiments are important to reduce risks and get feedback.
- Pilot projects and prototypes: Implement AI solutions as small pilot projects that allow for quick evaluation of results and learning of lessons.
- Data collection: Focus on collecting quality data and preparing it. We recommend analyzing data flows and removing irrelevant or inaccurate data.
Measurement: Set clear and action-oriented metrics that assess the effectiveness of the AI solution.
Scaling
We emphasize standardizing and continuously improving successful prototype solutions to ensure consistency and high quality.
- Standardization: Create standardized processes and best practices for implementing AI solutions. Lean IT recommends creating toolkits and guidelines that help ensure consistent implementation throughout the organization.
- Process integration: Ensure that AI solutions are smoothly integrated into existing processes and systems. According to Lean IT, the focus should be on automating processes and increasing efficiency without excessive complexity.
***
The Lean IT philosophy emphasizes that the journey of innovation and improvement is an ongoing process, with continuous improvement at its core. This approach is crucial for organizations aiming to stay competitive in today's rapidly changing business environment.
One key aspect of continuous improvement is adopting an iterative approach, which involves regularly collecting feedback from AI application users and analyzing the results to identify areas for enhancement. The PDCA (Plan-Do-Check-Act) cycle provides a structured framework for implementing this process, allowing organizations to plan, test, evaluate, and refine changes on a small scale before implementing them more broadly.
Effective change management principles are equally important. Clear, transparent communication ensures that all stakeholders understand the reasons behind the changes and their impact on daily work processes. Involving employees from all levels of the organization promotes a sense of unity and collective adoption of new technologies and practices. Providing ongoing training and support is essential to ensure that employees are well-equipped to handle the changes and make the most of the AI applications being implemented.
Continuous improvement does not always require major overhauls; Lean IT encourages organizations to make small, well-thought-out changes on a regular basis. This approach allows for a more gradual and manageable transition, reducing the risk of disruption to daily operations.
In addition to these principles, organizations must prioritize data quality and accessibility, enabling employees to leverage the full potential of AI applications and make informed decisions based on the insights generated. Risk management is also vital, and Lean IT recommends using structured tools and methods for identifying and addressing potential risks associated with AI applications.
By embracing the principles of continuous improvement and effective change management, organizations can foster a culture of innovation and adaptability. This approach enables companies to successfully navigate the complexities of AI implementation and harness the full potential of these transformative technologies.
Conclusion
Implementing artificial intelligence in an organization brings up several challenges and changes that require a careful approach. The responsibility of leaders is to deal not only with technical aspects but also to overcome human and cultural barriers. Fear of the unknown and resistance to change are natural phenomena that need to be handled carefully. Transparent communication, consistent feedback, and active employee involvement are key elements that help build trust and readiness to cope with changes.
Historically, organizations have been more successful with small, gradually introduced changes. Therefore, especially for complex innovations like AI, it's important to start with small pilot projects and use an iterative approach. Lean IT methodologies and continuous experimentation help reduce risks and ensure that AI implementation brings real value to the organization.
When managing changes, it's important to choose a suitable and effective framework, such as Kotter's 8-Step Model, the ADKAR model, or the McKinsey 7S Framework. These structured approaches help organizations manage change systematically and successfully.
Implementing AI in an organization is usually not a one-time event but a continuous and iterative process. There is always an opportunity to improve systems and processes and learn from experiences and feedback. This requires resilience and readiness from leaders to experiment with new solutions.
In summary, AI implementation is simultaneously a complex and opportunity-rich step that requires strategic thinking, openness, and an innovative approach from management.If a balance can be maintained between the adoption of new technologies and resource conservation, AI implementation can offer organizations a competitive edge and create higher value for both employees and customers. It's important that leaders understand human barriers and are able to provide support and transparent communication throughout the process.